The advent of advanced technologies is redefining industries and changing how businesses operate and the banking sector is not any different. The two technologies that are driving this digital revolution are artificial intelligence (AI) and machine learning (ML). These two are reshaping the way banks operate, interact with customers, and manage risk. A recent Deloitte survey revealed how 86% of financial services AI adopters say that AI will be very or critically important to their business’s success in the next two years. Why not? After all, with their capabilities to detect patterns, and process vast amounts of data faster with much accuracy, AI in banking is improving efficiency, speeding up processes, managing risk, and positively influencing customer experience.
So, what should banks do to keep current with AI marketplace trends and build confidence in the future? To reap the full benefits of new AI and ML in banking, financial service providers must move beyond the hype and consider the practical applications of AI in banking. Therefore, in this blog, we’ll explore seven key areas where AI and ML are transforming financial services. Additionally, we’ll also discuss how to integrate these technologies to fully realize their potential for your BFSI services.
7 Ways AI in Banking is Redefining & Enhancing Financial Services
What are ML and AI in Banking?
We have seen how the adoption of AI technologies is becoming a huge part of financial institutions. It’s helping BFSI to not only automate processes, and reduce operational costs but also enhance overall productivity. Meanwhile, traditional machine learning systems are widely utilized in areas such as fraud detection, and personalized marketing strategies, among others. Therefore, AI combines computer science and robust datasets to enable problem-solving, and ML, a sub-field of AI, enables custom software applications to make more accurate predictions after analyzing large volumes of data, reducing risk considerably for banks and financial institutions. So, let’s understand how banks are leveraging both technologies to their advantage:
- Personalized Customer Experiences
AI-powered chatbots and virtual assistants are revolutionizing customer interactions. These intelligent systems can understand and respond to complex queries, providing personalized financial advice, and offering tailored recommendations. For example, a virtual assistant can analyze a customer’s spending habits and suggest ways to save money or invest wisely. - Enhanced Fraud Detection and Prevention
By analyzing historical data and real-time transactions, AI systems can flag suspicious activities, such as unusual spending patterns or unauthorized access attempts. Therefore, machine learning algorithms help banks identify patterns in vast datasets, enabling banks to detect fraudulent transactions in real time. This proactive approach helps banks prevent financial losses and protect customer accounts. - Accurate Risk Assessment and Credit Scoring
Traditional credit scoring models often rely on limited data points, such as credit history and income. AI-powered risk assessment models can analyze a wider range of factors, including social media activity, alternative data sources, and behavioral patterns, to provide more accurate and comprehensive credit risk assessments. Thus, enabling banks to make more informed lending decisions and reduce the risk of default. - Improved Operational Efficiency
AI and ML can automate routine tasks, such as data entry, report generation, and customer service inquiries, and process vast amounts of data quickly and accurately, freeing up human resources to focus on more strategic initiatives. Additionally, this not only speeds up operations but also minimizes human error. For example, AI-powered robotic process automation (RPA) can automate repetitive tasks, reducing errors and increasing productivity. - Enhanced Cybersecurity Solutions
Banking services deal with customer’s sensitive financial data and with growing online threats and cyberattacks, it becomes imperative for banks to be more vigilant. In that scenario, AI-driven cybersecurity solutions can proactively identify and respond to cyber threats. By analyzing network traffic, user behavior, and other data sources, AI can detect anomalies and potential security breaches, such as phishing attacks and malware infections. Doing so enables banks to protect sensitive financial information and safeguard customer accounts. - Automated Trading Systems
Algorithmic trading powered by AI and ML enables banks to execute trades at high speed and with precision. AI, with its advanced algorithms and capabilities to analyze vast amounts of data, identify patterns, and make predictions at once unimaginable speeds. Therefore, AI algorithms are employed to process news feeds, market data, social media sentiment, and other relevant information, enabling them to predict market trends and execute trades automatically. Thus, banks utilizing AI-driven trading algorithms and systems can react to market events in milliseconds, giving banks a competitive edge. - Driving Financial Inclusion
There’s another advantage, more precisely, a social advantage of integrating AI and ML in banking services- that it can play a crucial role in expanding access to financial services for underserved populations. For example, AI can analyze mobile phone usage patterns, social media activity, and other digital footprints to assess creditworthiness, enabling individuals with limited credit history to access loans and other financial products. This has the potential to significantly improve financial inclusion and empower individuals and communities in the areas or communities that are underbanked and unbanked.
Build next-gen, data-driven financial solutions with AI and ML that offer smarter insight, optimize user experience, and enhance security.
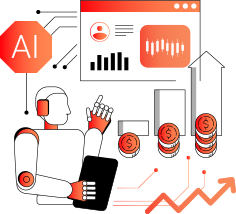
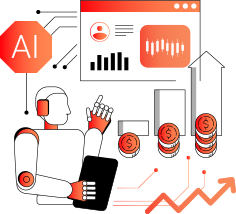
Integration of AI in Banking: Challenges and Opportunities Ahead
Major banks and financial institutions are moving to the next level with ML, natural language processing (NLP), and GenAI. Capital One has been using “Eno” a virtual assistant for personal banking, while PNC Financial Services Group offers a mobile banking platform “PINACLE” that has a cash forecasting feature that leverages AI and ML systems to make data-based predictions about a company’s financial future to inform decision making.
Additionally, 58% of banking CIOs in 2024 reported they had already deployed or are planning to deploy AI initiatives this year, while this figure will increase to 77% in 2025. Additionally, in a report by IDC, global annual spending on AI by banks and finance firms is expected to reach $64.03bn by 2030, with extra spending of $31bn on AI embedded in existing systems by 2025.
Despite the promising picture it presents, there are certain challenges to implementing AI in banking. Data privacy and security are paramount, as banks handle sensitive customer information. Additionally, issues with integrating AI with legacy systems can be technologically demanding and expensive. Other than that, there’s an issue of maintaining transparency and avoiding bias in AI algorithms that are critical to building trust and ensuring fair outcomes.
But all is not lost here, with proper AI strategy and the right collaboration, banking services providers can overcome these challenges. For instance, they can invest in robust data security measures and ensure compliance with regulations or to keep transparency and fairness in AI algorithms, proper training and workshops should be offered to help build trust and ensure ethical outcomes.
5 Tips to Implement AI and ML in Banking Systems: What You Need to Know
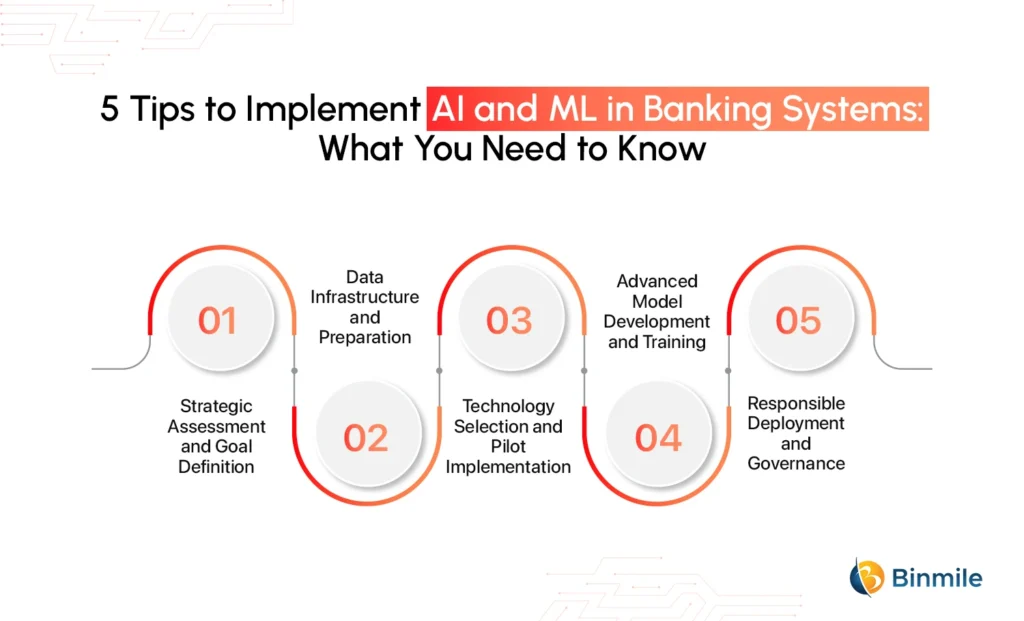
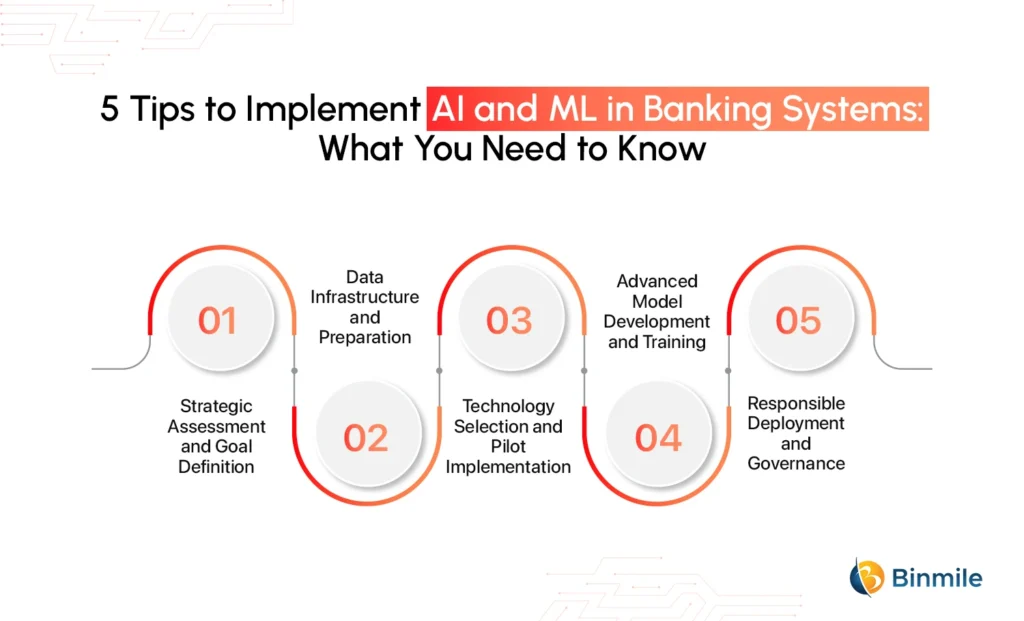
Now that we’ve looked into the real-world examples of AI in banking, and also understand the way AI and ML in banking are modernizing financial services, let’s dive into a few steps you can also integrate both technologies in your operations and systems:
- Strategic Assessment and Goal Definition
Banks must conduct a comprehensive analysis of existing processes to identify AI optimization opportunities. By assembling a cross-functional team of experts from IT, data science, and strategy, organizations can establish clear, measurable objectives. The focus should be on defining specific goals that align with the bank’s strategic vision, such as improving customer experience or reducing operational costs. - Data Infrastructure and Preparation
Developing a robust data management strategy is crucial for successful AI implementation. Banks must audit existing data sources, ensure regulatory compliance, and create sophisticated data pipelines. This involves investing in secure infrastructure, implementing data anonymization techniques, and preparing high-quality, integrated data that can support advanced machine learning and artificial intelligence applications. - Technology Selection and Pilot Implementation
Banks should carefully evaluate and select AI platforms that match their specific technological requirements. The recommended approach involves initiating focused pilot projects in strategic areas like fraud detection or customer service. Using cloud-based ML platforms, organizations can develop proof-of-concept projects with clear success metrics, allowing controlled testing and validation of AI technologies. - Advanced Model Development and Training
Machine learning model development requires employing diverse techniques such as supervised and unsupervised learning. Banks must train models on extensive historical data, creating sophisticated systems for predictive analytics and complex pattern recognition. Rigorous testing, ensemble modeling, and establishing continuous learning mechanisms are essential to develop accurate, adaptable AI solutions. - Responsible Deployment and Governance
Implementing a comprehensive governance framework is critical for responsible AI deployment. Banks must ensure transparency, develop ethical guidelines and maintain human oversight in critical decisions. This involves creating monitoring systems, addressing potential biases, protecting customer privacy, and investing in employee training to effectively integrate AI technologies into existing banking operations.
60% of financial services companies have implemented at least one AI capability, don’t stay behind! Empower your business today with tailored AI solutions.
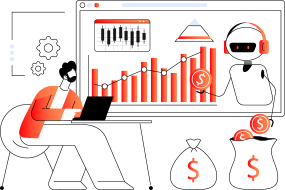
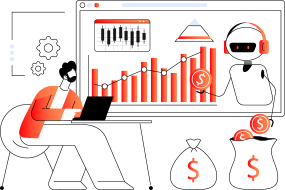
Sum It Up
Artificial intelligence is set to accelerate growth and bring digital transformation across the banking sector. Moreover, enabling these financial platforms to adopt new sales strategies, improve efficiency, focus on data utilization, and offer personalized services and interactions on a large scale. This is the reason, ML and AI in banking are no longer futuristic concepts and are driving and redefining the modern financial services industry. As AI and ML continue to evolve, we can expect even more innovative applications in the future. Therefore, the future of AI in banking is bright and promising, however, banks need to understand the advantages and challenges these two bring for successful implementation.
Being a leading FinTech software development services company we can help offer AI-driven digital solutions and develop custom AI and ML-based models to help you streamline processes, reduce errors, mitigate risks, and enhance customer experience. Our AI experts have years of experience in the financial industry and can assist you in utilizing AI to generate transformational changes, so get in touch today with them about AI-related consultancy and development services.